News & Updates
Program News
Our Georgetown University Health Informatics and Data Science (HIDS) program has been listed among the top 20 Health Data Science Degrees by the Healthcare Management Degree Guide .
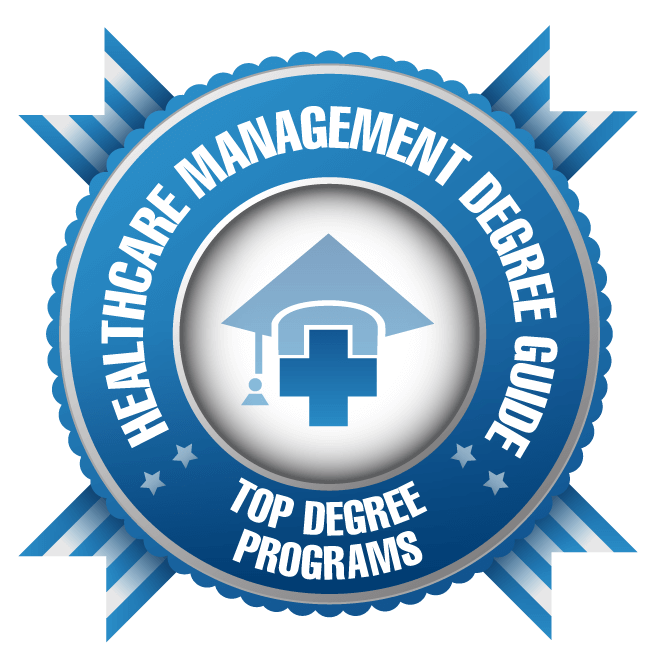
We are proud to announce that the Georgetown Health Informatics and Data Science (HIDS) program is featured among the best value schools for masters degrees in Health Informatics & Data Science by BestValueSchools in 2021 and 2022 ! https://lnkd.in/djshqdB
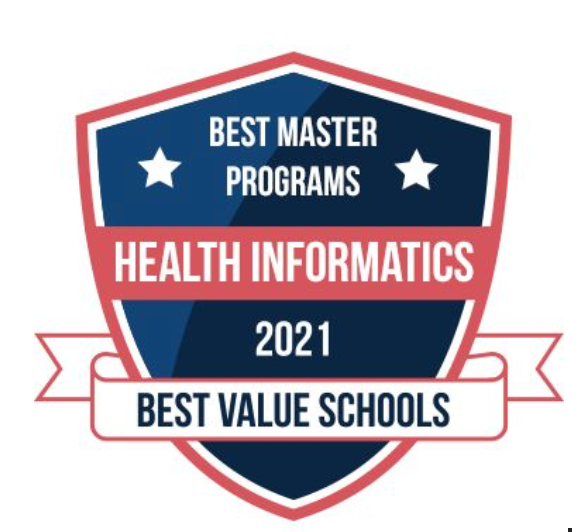
Our YouTube channel is up for our Georgetown University Health informatics & Data Science (HIDS) master’s program. Check out our short infomercials as well as longer videos of our STEM-based program, info session and our SevenBridges webinar.
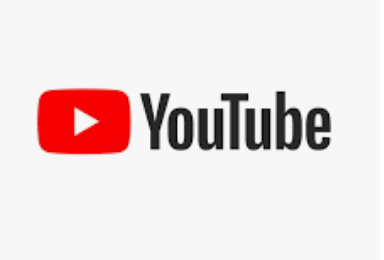
Read our latest paper in Nature Communications published in December 2022 regarding a large scale consortium effort to develop a federated learning approach for AI models based on brain cancer imaging. The segmented labels from our REMBRANDT MRI dataset are part of a world-wide federated platform Federated Tumor Segmentation (FeTS) https://www.fets.ai/ that allows training specific machine learning models by leveraging information gathered from brain cancer datasets residing in collaborating sites without ever exchanging the data. Such a world wide platform enables very large multi-site machine learning models in an effort to accelerate discovery. In this paper, we describe how this Federated Learning model enabled big data for Rare Cancer Boundary Detection. This work was published with our collaborators Sypiron Bakas, Sarthak Pati, Ujjwal Baid et al at UPenn. Read a short summary of the paper here .
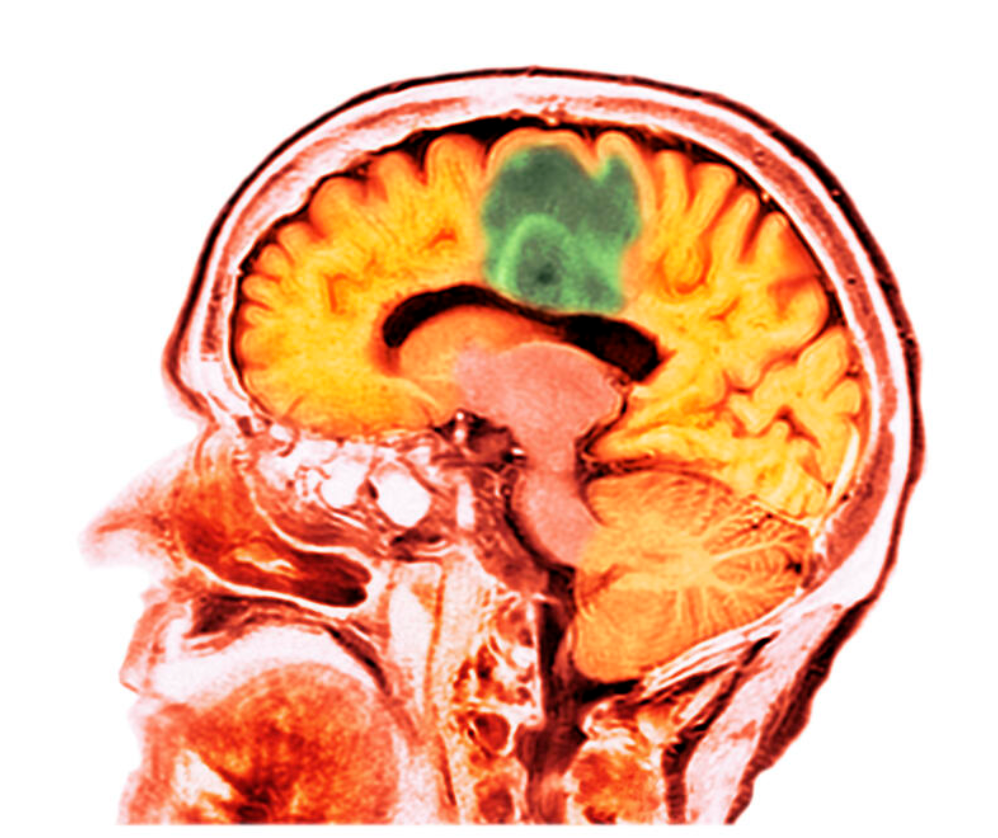